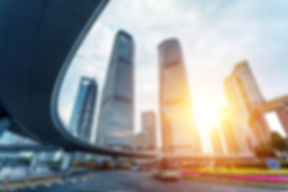



Intro to Statistical Learning
This course introduces descriptive statistics, probability, and statistical inference. Participants will learn the difference between population parameters and the statistics from samples that estimate them. Participants will be able to use summary statistics to describe distributions and answer business related questions. Participants will also learn statistical inference and prediction (hypothesis tests, confidence intervals) and how to use these methods to solve business problems.
Course Objectives
After completing this course, participants will be able to:
-
Explain the different types of modeling problems and methods, including supervised versus unsupervised learning and regression versus classification
-
Explain the common methods of assessing model accuracy
-
Employ basic methods of exploratory data analysis, including data checking and validation
-
Use statistical inference to estimate (using confidence intervals) and test (hypothesis tests) the population means
-
Comprehend common probability distributions and have the ability to select the appropriate distribution for business problems
-
Calculate correlation coefficients and understand when to use the appropriate coefficient (Spearman vs Pearson)
-
Design experiments that have sufficient statistical power to calculate an effect of interesting size

Module 1: Descriptive Statistics
-
Statistical measures of centrality (mean, median, mode)
-
Statistical Measures of spread (quartiles, variance, standard deviation)
-
Measures of relationships (correlation, scatter plots, two-way tables)
-
Graphical representations of data (frequency tables, histograms, box plots)
-
Sampling and the role of normality
-
Working with skewed distributions

Module 2: Probability
-
Discrete distributions and the probability mass function
-
Continuous distributions and the probability density function
-
Cumulative probability distributions
-
Expected values of distributions
-
Common discrete distributions (Uniform, Bernouli, Binomial, Poisson)
-
Common continuous distributions (Exponential, Normal, Students’ t, Chi-Square)
-
Baye’s Theorem
-
Central Limit Theorem and law of large numbers

Module 3: Inferential Statistics
-
Correlation vs. causation
-
Confidence intervals and p-values
-
Introduction to hypothesis testing: distinguishing between Type I and Type II error
-
Performing two-sample t-tests
-
Multiple comparisons and Bonferroni adjustments
-
False Discovery Rate
-
Power and sample size calculations
-
Design of experiments
-
Chi-square tests for categorical data